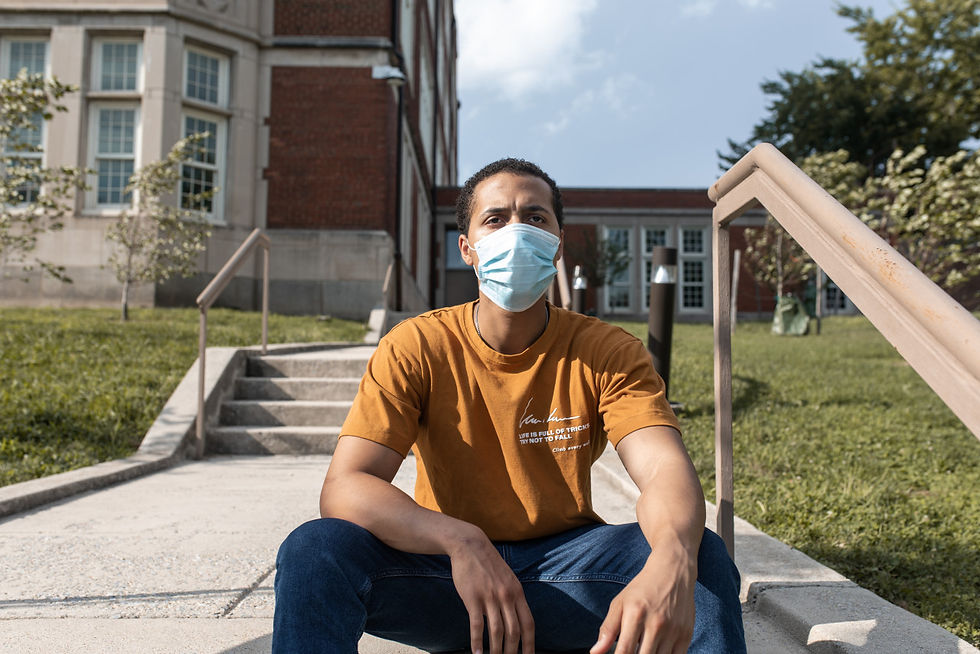
Artificial intelligence (AI) – the technology that helps machines think like humans – is everywhere. Whether we willingly yield ourselves to it, or it seduces us into submission, AI is actively involved in our everyday lives. This statement has become increasingly apparent during the COVID-19 global pandemic where complicated debates around privacy, access, and the weaponization of Big Data have become mainstream. In this article, I argue there is no equivalent to the Fair Housing Act for the algorithms and surveillance tools used in housing decisions. Instead, these technologies serve as tools to displace people who are already usually disadvantaged. Moreover, while housing authorities, financing agencies, and property owners in the United States are to be held accountable to the Fair Housing Act, this is more easily said than done. I conclude that, as housing practices and decisions are continually moved to machines, they too must be subject to not violating individual civil rights and liberties.
To begin, let’s ground ourselves with helpful definitions. The Fair Housing Act of 1968 prohibited discrimination concerning the sale, rental and financing of housing based on race, religion, national origin or sex.” This piece of legislation was extremely important for Black, Native, Asian, and non-White Hispanic people—all communities that are less likely to achieve homeownership.
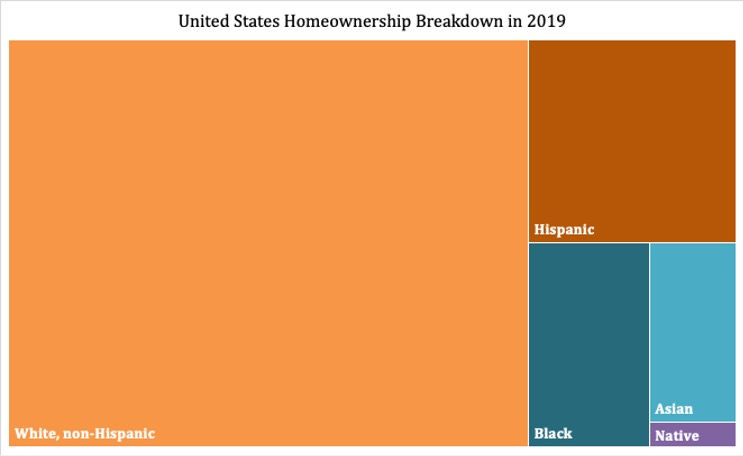
Research has demonstrated that landlords subject tenants of colour to eviction proceedings at higher rates than white tenants, even when controlling for all other factors. Though the Fair Housing Act had measured intentions to allow for more fair housing practices, it was not a sweeping solution. Not only do non-white groups have lower homeownership rates than their white counterparts, they also own fewer homes than white people due to non-white groups composing less than 40 percent of the U.S. population. When it comes to civil rights protections, the fair and consistent enforcement of these policies is even more important than the policies themselves.
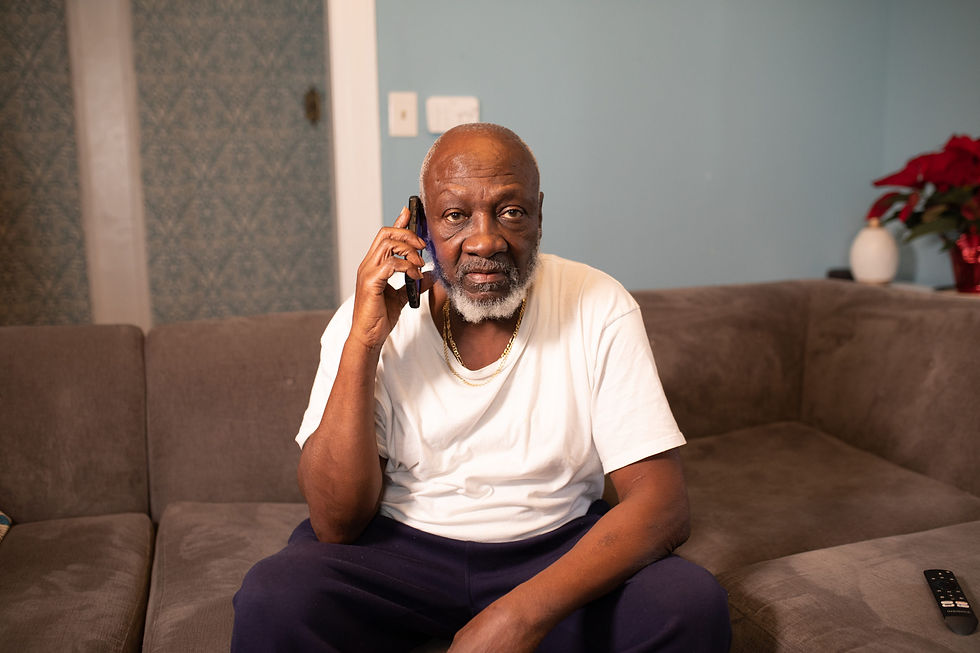
The story gets even more complicated when we introduce AI into housing decisions. IBM offers a useful description of AI: “Artificial intelligence leverages computers and machines to mimic the problem-solving and decision-making capabilities of the human mind.” While this definition makes the concept of AI seem straightforward, there are underlying factors complicating this work theoretically and practically. The challenge as scholars such as Ruha Benjamin, Joy Buolamwini, Timnit Gebru, Safiya Noble, and Jennifer Eberhardt have discussed in their research. Thus, these algorithms aren’t absolved from perpetuating biases. We already know people can be negatively biased, so this raises the question: why would we want to mimic the capabilities of the human mind? Wouldn’t we instead want to use AI to create a model of problem-solving and decision-making far superior in ethical conviction, empathy, and reason than what we humans can achieve on our own?
In 2015, a Supreme Court ruling in the case of Texas Dept. of Housing and Community Affairs v. Inclusive Communities Project affirmed that disparate impact claims could be used as legal tools in FHA cases. As Google Arts & Culture explains, disparate impact refers to the “practices in employment, housing, and other areas that adversely affect one group of people of a protected characteristic more than another, even though rules applied by employers or landlords are formally neutral”. This convention means that even if explicit discriminatory intent is absent, it can be inferred from the impact of these practices. This changed, however, as the September 2020 final rule from the U.S. Department of Housing and Urban Development (HUD) effectively absolved housing providers of AI-related disparate impact liability if they asserted that their work relied on algorithmic models. Not only is this decision a flagrant step backwards for civil rights, but it is in direct contradiction to existing research on AI and the accountability needed from its developers and users.
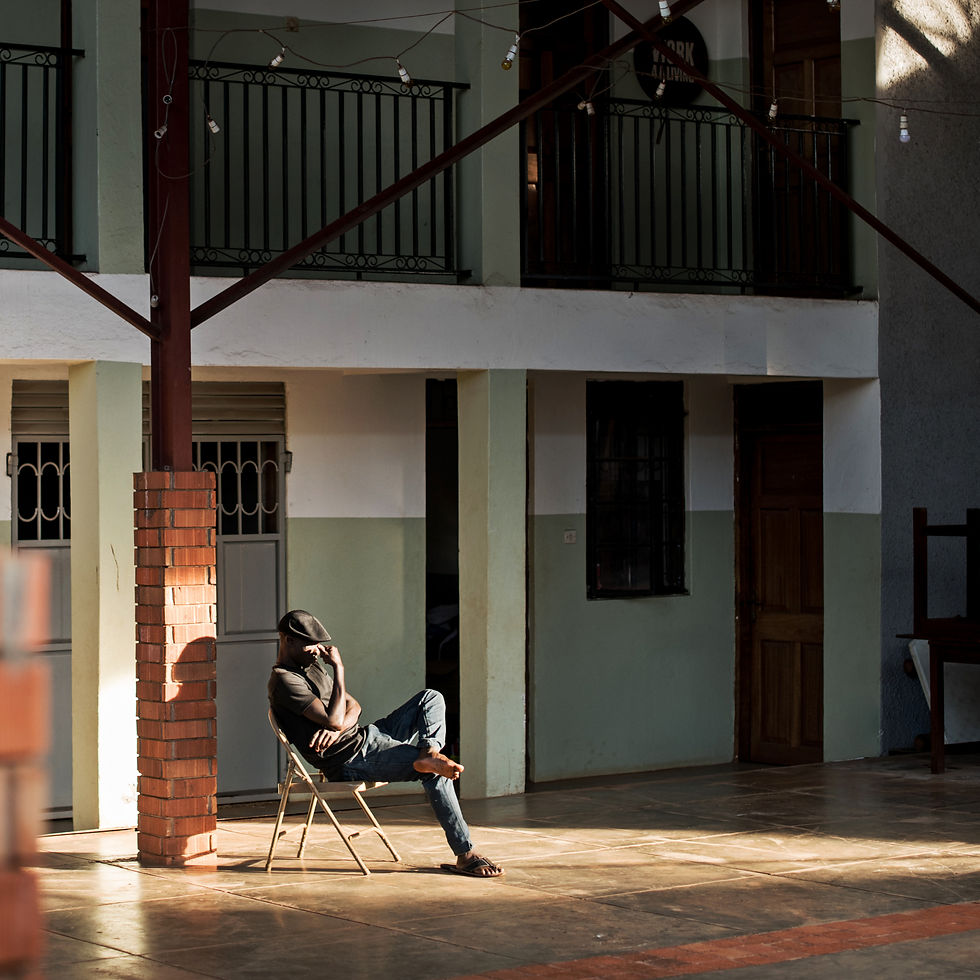
It can be easy to assume that, because algorithms employ mathematical principles, they’re objective and thus don’t reinforce racial realities; the opposite is true. In AfroTech’s reporting on Bloomberg’s analysis of federal mortgage data, it was revealed that in 2020 Wells Fargo rejected 53% of Black refinance applications compared to only 28% of white refinance applications. These figures are real people, including one Black engineer with an above-800 credit score and a doctor as a spouse. The denial of two seemingly-exceptional candidates helps to question Wells Fargo’s assertion that all applicants are treated equally and their defence that such disparities were caused by “additional, legitimate, credit-related factors”. Moreover, the reality that more lower-income white applicants were approved than the highest earning Black applicants is further evidence.
A major challenge is the lack of protection afforded to the common people who are impacted by these algorithms. As there is insufficient enforcement of the Fair Housing Act, communities of colour are still disadvantaged in the housing market. These risks threaten the rights to housing, privacy, security and other basic human rights for these potential homebuyers. Now that we see how this system has failed, where do we go from here?
Equitable algorithms: The MIT-IBM Watson AI Lab scholars have endorsed the peer-reviewed Distributionally Robust Fairness (DRF) and SenSR training algorithm, which depends on the EXPLORE algorithm to learn a fair metric from the data. As recommended, this methodology must now be validated in real world housing algorithms.
Enforced accountability: Lenders should be held responsible for their role in leveraging algorithms to institutionalize the practice of discriminating against Black neighbourhoods (redlining). Lenders must also be accountable for targeting minority groups whose financial prospects have been most undermined by structural and cultural prejudice through credit they can’t afford (reverse redlining).
Education and competency: Those preparing, developing, deploying, using, and subject to these algorithms must be knowledgeable of how these data and algorithms work. Creating just algorithms will require a collective co-creation and co-review effort.
Effective policy: The Fair Housing Act should not be the standard; instead policies and practices that more aggressively lead to equitable realities should be developed.
Myesha Jemison [2021] investigates artificial intelligence and inequity, indigenous knowledge and power, and settler colonialism and technology. She’s founder of Scholourship Magazine and writes Afro-indigenous futurist fiction.
Коментарі